What is a Scatterplot in ABA?
Unveil the power of a scatterplot in ABA! Learn to decode behavioral patterns and trends effortlessly.


Introduction to ABA and Scatterplots
When delving into the realm of Applied Behavior Analysis (ABA), a fundamental tool utilized is the scatterplot. Understanding the basics of ABA and the role of scatterplots is key to deciphering behavioral patterns and trends effectively.
Understanding Applied Behavior Analysis (ABA)
Applied Behavior Analysis (ABA) is a scientific approach aimed at understanding and modifying behavior. It involves the systematic application of behavioral principles to analyze and address behavioral challenges. ABA is widely used to improve social skills, communication, learning, and daily living skills in individuals across various age groups.
What is a Scatterplot in ABA?
In the context of ABA, a scatterplot is a visual representation of the relationship between two variables. Typically, one variable is plotted on the X-axis (independent variable) and the other on the Y-axis (dependent variable). By plotting data points on a scatterplot, analysts can identify patterns, trends, and correlations within the data, providing valuable insights into behavior and intervention effectiveness.
Understanding the role and significance of scatterplots in ABA is crucial for practitioners and researchers alike, as they serve as powerful tools for data visualization and analysis in behavior modification and intervention planning.
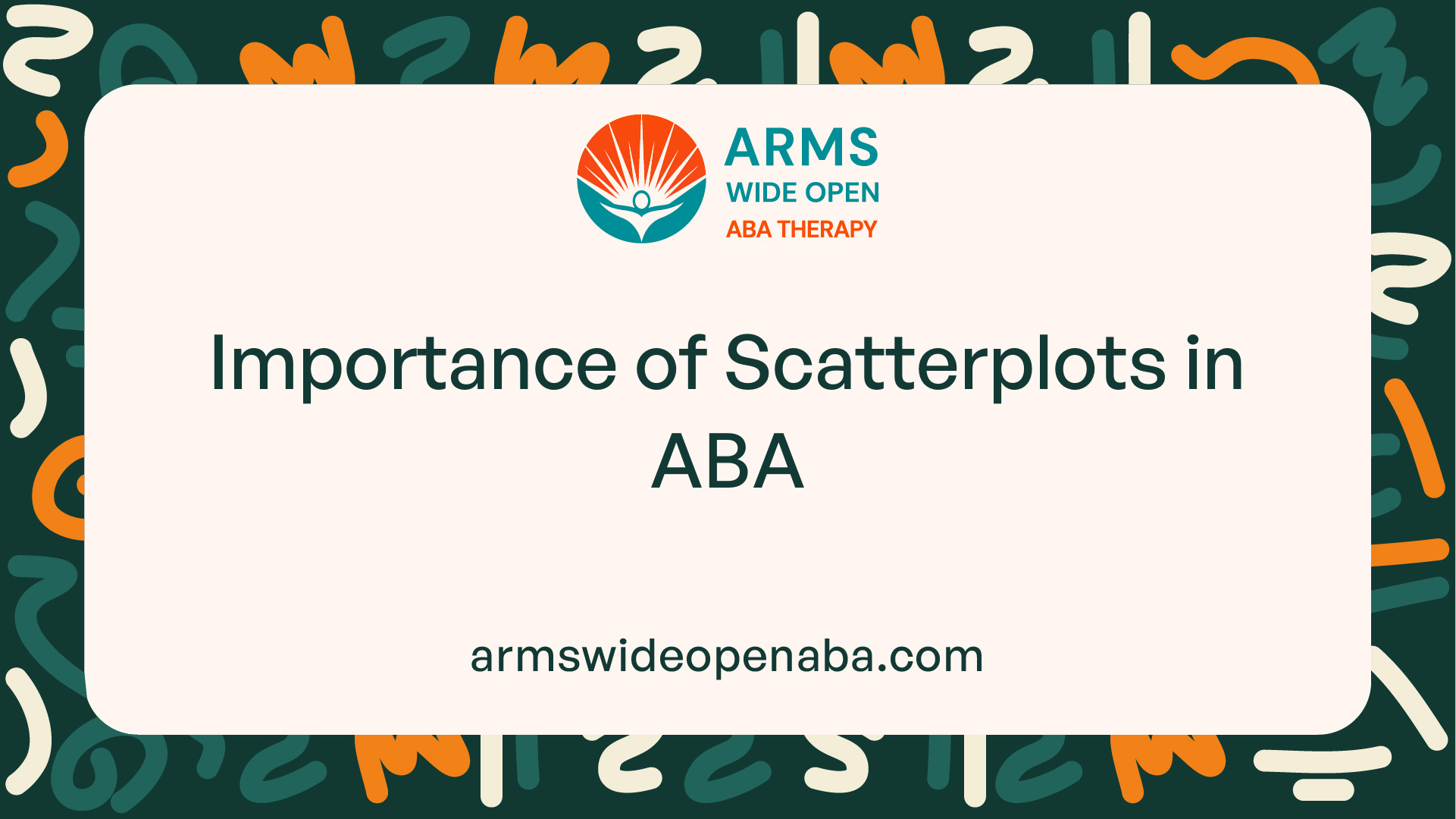
Importance of Scatterplots in ABA
In the realm of Applied Behavior Analysis (ABA), scatterplots play a pivotal role in deciphering and understanding behavioral patterns. By examining scatterplots, analysts can effectively identify patterns and trends, allowing for a comprehensive analysis of behavioral data.
Identifying Patterns and Trends
One of the primary functions of scatterplots in ABA is their ability to visually represent data points, making it easier to identify trends and patterns within behavioral data. By plotting independent and dependent variables on a graph, analysts can quickly discern correlations, clusters, or outliers that may not be apparent when looking at raw data alone.
Scatterplots provide a visual roadmap that aids in recognizing consistent trends or irregular behaviors over time. This visual representation enhances the efficiency of data analysis, enabling practitioners to spot recurring behavioral patterns and make informed decisions based on the observed trends.
Monitoring Behavioral Changes
Another key benefit of utilizing scatterplots in ABA is the capability to monitor behavioral changes effectively. By tracking changes in behavior over time through scatterplots, analysts can visualize the progress or regression of behaviors in response to various interventions or environmental factors.
Through the use of scatterplots, analysts can track the effectiveness of behavioral interventions and assess whether the implemented strategies are yielding the desired outcomes. This ongoing monitoring of behavioral changes using scatterplots allows for timely adjustments to interventions and the development of targeted strategies to address specific behavioral goals.
In summary, the importance of scatterplots in ABA lies in their ability to unveil hidden patterns, trends, and behavioral changes within data sets. By leveraging scatterplots as a visual tool, analysts can gain valuable insights into behavior, facilitate decision-making processes, and drive more effective behavior intervention planning within the realm of Applied Behavior Analysis.
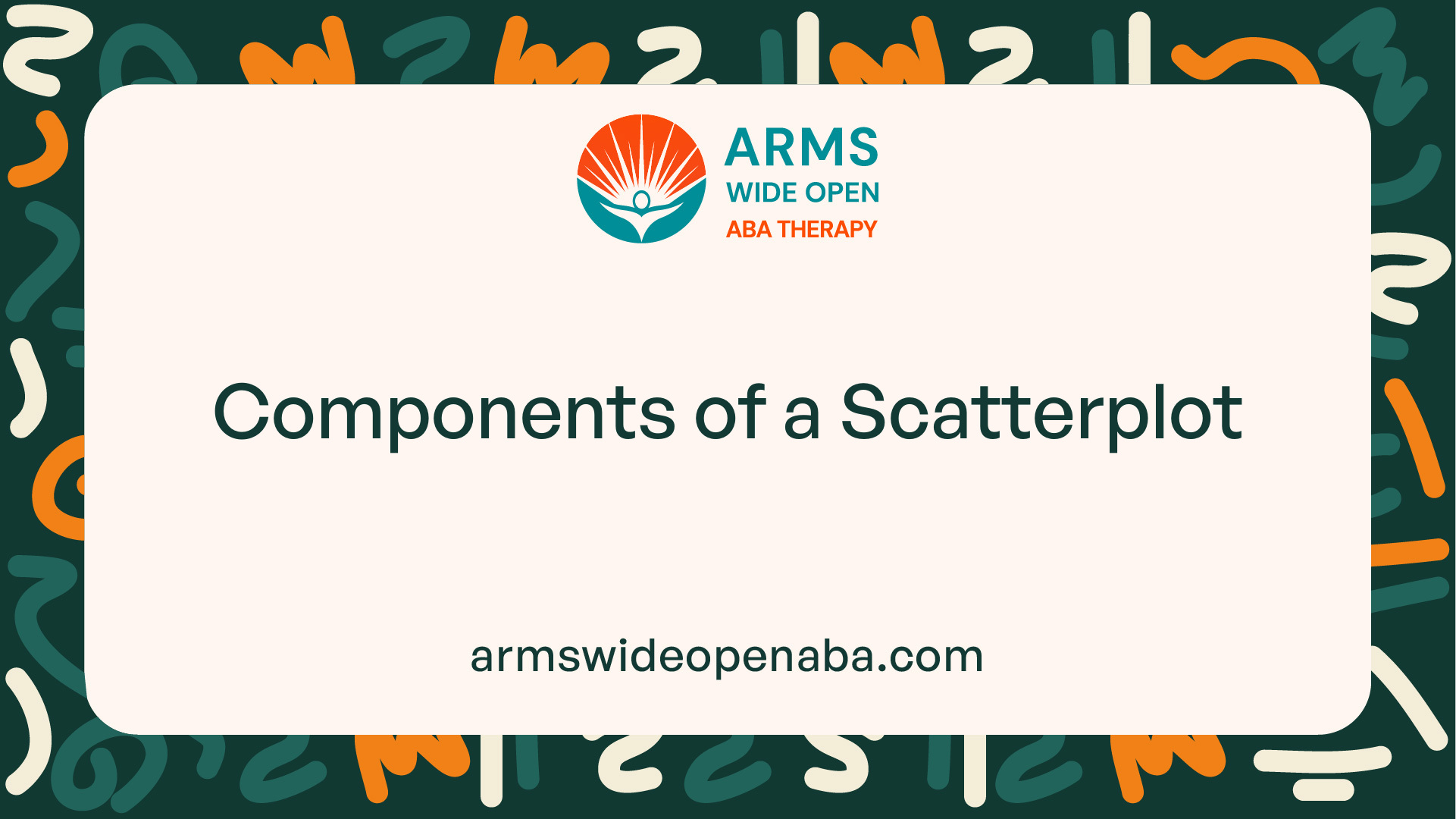
Components of a Scatterplot
When it comes to understanding the components of a scatterplot in Applied Behavior Analysis (ABA), it is essential to grasp the fundamental elements that make up this graphical representation of data.
X-Axis: Independent Variable
In a scatterplot, the X-axis represents the independent variable, which is the factor that is manipulated or controlled in a study. This variable is plotted along the horizontal axis and is not influenced by other variables in the analysis. The independent variable is typically displayed in a systematic and ordered manner to facilitate the interpretation of relationships within the data.
Y-Axis: Dependent Variable
Conversely, the Y-axis of a scatterplot represents the dependent variable, which is the outcome or response that is being measured or observed. This variable is plotted along the vertical axis and is influenced by changes in the independent variable. By plotting the dependent variable against the independent variable, researchers can visualize the relationship between the two variables and identify any patterns or trends that may exist.
Data Points and Patterns
The data points on a scatterplot are individual observations or measurements that correspond to specific values of the independent and dependent variables. Each data point is represented by a symbol, typically a dot, on the graph. By examining the distribution of data points on the scatterplot, researchers can identify patterns, trends, and relationships between the variables under investigation.
Utilizing the X-axis to represent the independent variable and the Y-axis to represent the dependent variable, scatterplots serve as a powerful tool in visualizing and analyzing data in ABA. By understanding these key components, researchers can effectively interpret the relationships between variables and draw meaningful conclusions from the graphical representation of data.

How to Create a Scatterplot
In the realm of Applied Behavior Analysis (ABA), creating a scatterplot is a fundamental tool for visualizing data and identifying patterns that can inform behavior interventions. The process of creating a scatterplot involves three key steps: the data collection process, plotting data points, and interpreting the scatterplot.
Data Collection Process
Before diving into creating a scatterplot, it is essential to gather accurate and relevant data. In ABA, data collection typically involves recording observations of behaviors over time. This data is usually collected in a systematic and consistent manner to ensure its reliability and validity.
To create a scatterplot, the data collected should include two variables: an independent variable (X-axis) and a dependent variable (Y-axis). The independent variable represents the factor that is manipulated or controlled, while the dependent variable is the behavior that is being observed and measured.
Plotting Data Points
Once the data has been collected and organized, the next step is to plot the data points on a graph. The independent variable is represented on the horizontal X-axis, while the dependent variable is shown on the vertical Y-axis. Each data point represents a specific observation or measurement of the behavior being studied.
By plotting the data points on the scatterplot, patterns and trends in the behavior can be visually identified. The arrangement of the data points can provide insights into the relationship between the independent and dependent variables and help in understanding how changes in one variable may affect the other.
Interpreting the Scatterplot
Interpreting the scatterplot involves analyzing the distribution of data points and identifying any correlations or trends that may exist. One of the key aspects of interpreting a scatterplot is examining the overall pattern of the data points. Are the points clustered together, forming a clear trend line, or scattered randomly across the graph?
Additionally, outliers or anomalies in the data should be noted, as they may indicate unique or exceptional observations that warrant further investigation. Understanding the implications of these outliers can provide valuable insights into the behavior being studied and guide the development of effective interventions.
By mastering the process of creating a scatterplot and interpreting the data it presents, behavior analysts can unlock the power of visual representation in ABA. Scatterplots serve as a dynamic tool for understanding behavior patterns, monitoring changes over time, and guiding interventions aimed at promoting positive behavioral outcomes.
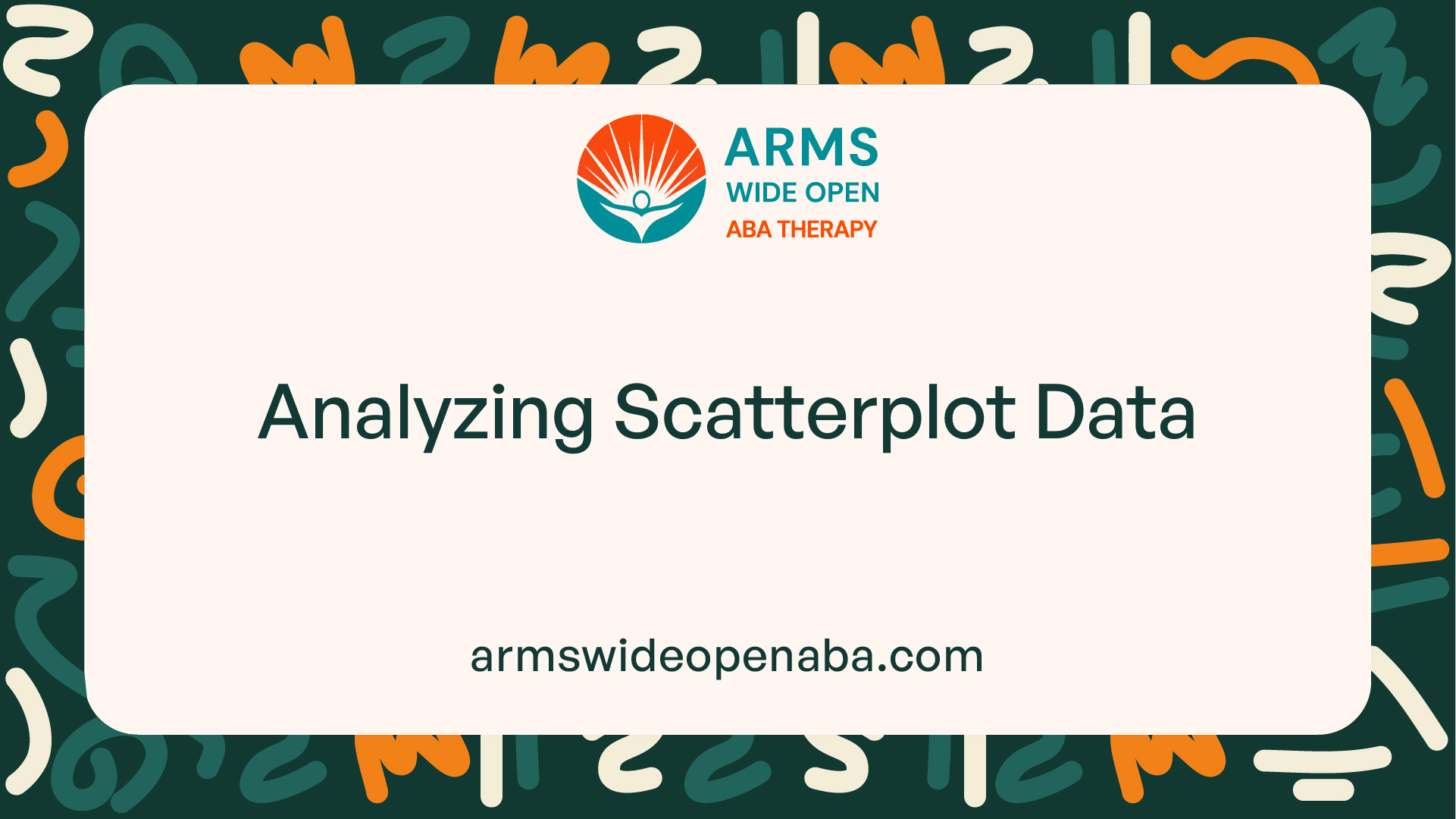
Analyzing Scatterplot Data
When it comes to analyzing scatterplot data in Applied Behavior Analysis (ABA), several key aspects come into play. Understanding the trends, correlations, outliers, and anomalies within a scatterplot provides valuable insights for behavior analysis and intervention planning.
Trend Lines and Correlations
Trend lines in a scatterplot help identify the direction and strength of the relationship between the independent and dependent variables. A positive trend line indicates a positive correlation, meaning that as one variable increases, the other also increases. Conversely, a negative trend line suggests a negative correlation, where one variable decreases as the other increases. Analyzing these trends allows behavior analysts to make informed decisions about interventions and strategies.
Outliers and Anomalies
Outliers and anomalies are data points that fall outside the normal pattern of the scatterplot. These points can significantly impact the overall interpretation of the data. Identifying outliers is crucial in ABA, as they may represent significant behavioral changes or atypical responses to interventions. By examining outliers closely, behavior analysts can gain insights into factors that may be influencing the individual's behavior.
Implications for Behavior Analysis
Analyzing scatterplot data offers crucial insights into behavior patterns, trends, and response to interventions. Understanding the implications of the scatterplot data is essential for developing effective behavior intervention plans, evaluating the effectiveness of interventions, and targeting specific behavioral goals. Behavior analysts use the information derived from scatterplot analysis to make evidence-based decisions that support the individual's behavioral progress and well-being.
By delving into trend lines, correlations, outliers, and anomalies within scatterplot data, behavior analysts can effectively interpret and leverage the power of visual representation in ABA. These analytical tools provide a comprehensive framework for assessing behavioral changes, identifying patterns, and guiding intervention strategies tailored to individual needs and goals.
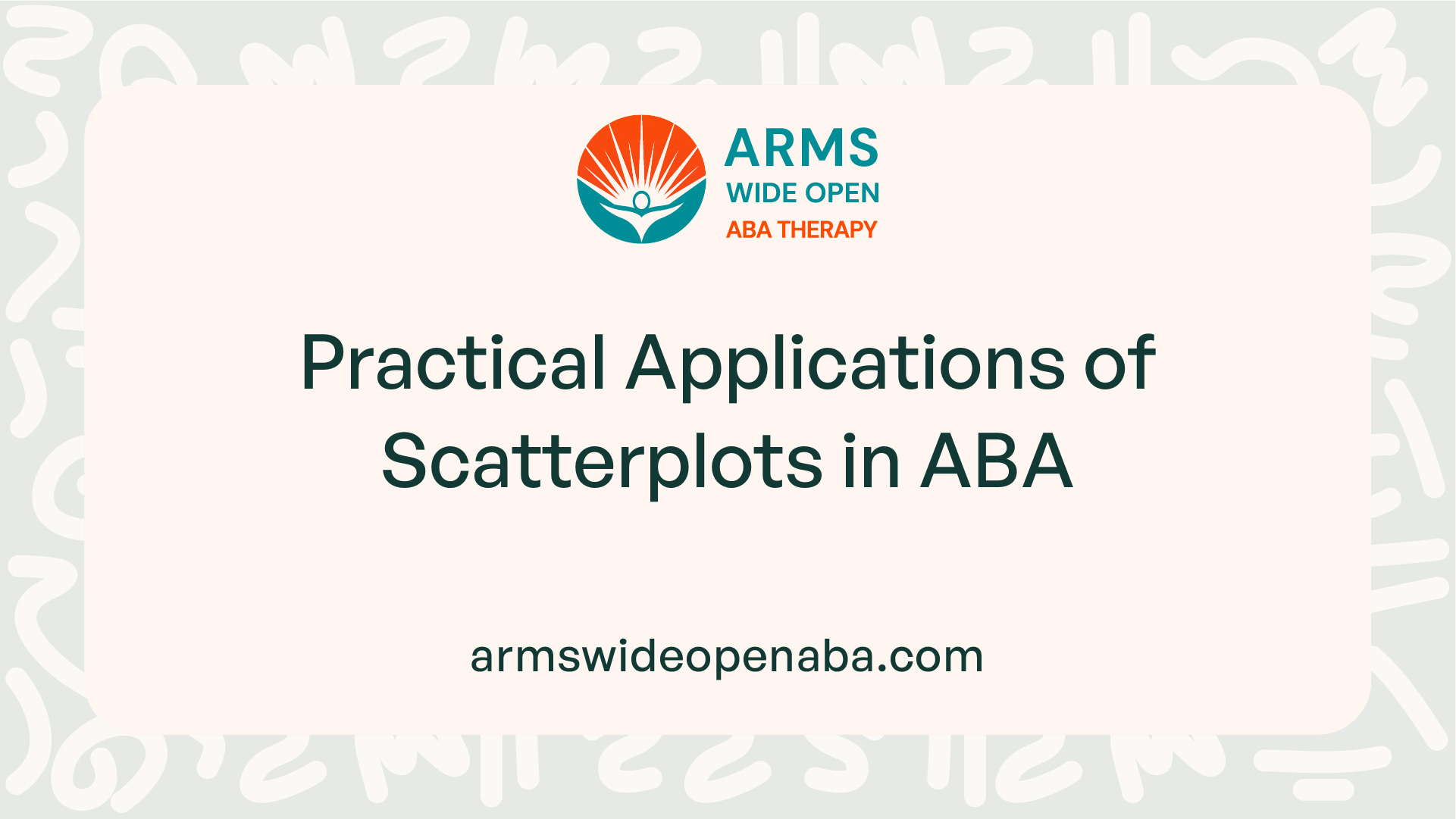
Practical Applications of Scatterplots in ABA
When it comes to Applied Behavior Analysis (ABA), scatterplots play a crucial role in several practical applications aimed at behavioral intervention and monitoring. Let's delve into three key practical applications of scatterplots in ABA: behavior intervention planning, evaluating the effectiveness of interventions, and targeting specific behavioral goals.
Behavior Intervention Planning
One of the primary applications of scatterplots in ABA is in the formulation of behavior intervention plans. By visually representing the relationship between the independent and dependent variables on a scatterplot, behavior analysts can identify patterns, trends, and correlations that inform the development of tailored intervention strategies.
Analyzing the data points on a scatterplot allows behavior analysts to pinpoint specific behaviors that may require targeted interventions. This data-driven approach ensures that behavior intervention plans are customized to address the individual needs and challenges of each client, leading to more effective outcomes.
Evaluating Effectiveness of Interventions
Another valuable application of scatterplots in ABA is in evaluating the effectiveness of behavioral interventions over time. By plotting data points before and after the implementation of an intervention, behavior analysts can visually assess any changes in behavior trends and patterns.
Comparing scatterplots before and after an intervention provides valuable insights into the effectiveness of the intervention strategy. Behavior analysts can identify positive shifts, trends, or correlations that indicate the intervention's impact on the targeted behaviors. This visual representation helps in gauging whether the intervention is achieving the desired outcomes or requires adjustments.
Targeting Specific Behavioral Goals
Scatterplots in ABA can also be utilized to target specific behavioral goals for clients. Behavior analysts can set measurable objectives based on the data patterns observed in the scatterplot, establishing clear targets for behavior change.
By identifying outliers or anomalies in the scatterplot data, behavior analysts can prioritize interventions for behaviors that require immediate attention. This targeted approach ensures that interventions are focused on addressing specific behavioral goals, leading to more efficient and effective behavior modification strategies.
By utilizing scatterplots in ABA for behavior intervention planning, evaluating intervention effectiveness, and targeting specific behavioral goals, behavior analysts can apply a data-driven approach to enhance the outcomes of behavior analysis and intervention strategies. These visual representations of data points provide valuable insights into behavioral patterns and trends over time, allowing practitioners to make informed decisions about treatment modifications. Scatterplots are particularly useful in identifying correlations between different variables, such as environmental factors and behavior frequency, which can lead to more precise and effective interventions. The visual nature of scatterplots makes them an especially powerful tool for identifying patterns that might not be immediately apparent in raw numerical data. This visualization capability becomes particularly crucial when dealing with complex behavioral cases where traditional data analysis methods might miss subtle but significant patterns.
Furthermore, the systematic collection and analysis of data through scatterplots enables behavior analysts to track progress objectively, adjust intervention strategies as needed, and communicate findings clearly with other professionals and stakeholders. This evidence-based approach not only improves the quality of behavioral interventions but also helps ensure that treatment decisions are based on concrete data rather than subjective observations alone. The ability to present data visually also facilitates better communication with parents, teachers, and other caregivers who may not have extensive training in behavioral analysis but need to understand the progress being made. This improved communication often leads to better buy-in from stakeholders and more consistent implementation of intervention strategies across different settings.
The implementation of scatterplot analysis in behavior intervention requires careful attention to detail and consistent data collection methods. Behavior analysts must establish clear protocols for recording behavioral incidents, including precise timing, duration, and intensity measurements. This level of detail allows for more nuanced analysis and helps identify subtle patterns that might otherwise go unnoticed. Additionally, when multiple team members are involved in data collection, having standardized procedures ensures consistency and reliability in the data gathered. Regular training and monitoring of data collection procedures help maintain the integrity of the data and the validity of any conclusions drawn from it. The development of comprehensive training materials and ongoing support for data collectors is essential for maintaining high-quality data collection practices.
One of the key advantages of using scatterplots is their ability to reveal temporal patterns and cyclical behaviors. For instance, they can highlight whether certain behaviors occur more frequently during specific times of day, days of the week, or in response to particular environmental triggers. This information is invaluable for developing targeted interventions that address behavior challenges at their source. Moreover, scatterplots can demonstrate the effectiveness of different intervention strategies by showing clear visual evidence of behavior change over time, making it easier to identify which approaches are most successful for individual clients. The ability to identify these patterns allows for more proactive intervention strategies and better resource allocation in behavior management programs.
The versatility of scatterplots extends beyond basic behavior tracking to more complex analyses. They can be used to examine the relationship between multiple variables simultaneously, such as the interaction between medication timing, environmental factors, and behavioral outcomes. This multi-dimensional analysis capability makes scatterplots an essential tool for behavior analysts working with complex cases or in situations where multiple interventions are being implemented concurrently. The ability to visualize these relationships helps in fine-tuning intervention strategies and making data-driven decisions about treatment modifications. Advanced statistical analyses can be applied to scatterplot data to reveal deeper insights into behavioral patterns and intervention effectiveness.
Modern technology has significantly enhanced the utility of scatterplots in behavioral analysis. Digital tools and software applications now allow for real-time data collection and immediate visualization, making it easier than ever to track and analyze behavioral patterns. These technological advances have also made it possible to share data and analyses more efficiently among team members and stakeholders, leading to more collaborative and effective intervention planning. The integration of mobile applications and cloud-based platforms has revolutionized how behavior analysts collect, analyze, and share data, making the process more streamlined and accessible.
In conclusion, the use of scatterplots in behavioral analysis represents a cornerstone of evidence-based practice. Their ability to clearly display behavioral patterns, intervention effects, and variable relationships makes them an indispensable tool for behavior analysts seeking to provide the most effective interventions possible. As technology continues to advance, the integration of digital tools for creating and analyzing scatterplots will likely further enhance their utility in behavioral intervention planning and implementation. The ongoing evolution of data analysis techniques and visualization tools promises to make scatterplots even more valuable in the future of behavioral analysis and intervention.
Sources
https://www.crossrivertherapy.com/aba-therapists/scatterplot
https://www.abtaba.com/blog/scatterplot-in-aba
https://www.bridgecareaba.com/blog/scatterplot-in-aba
Similar articles
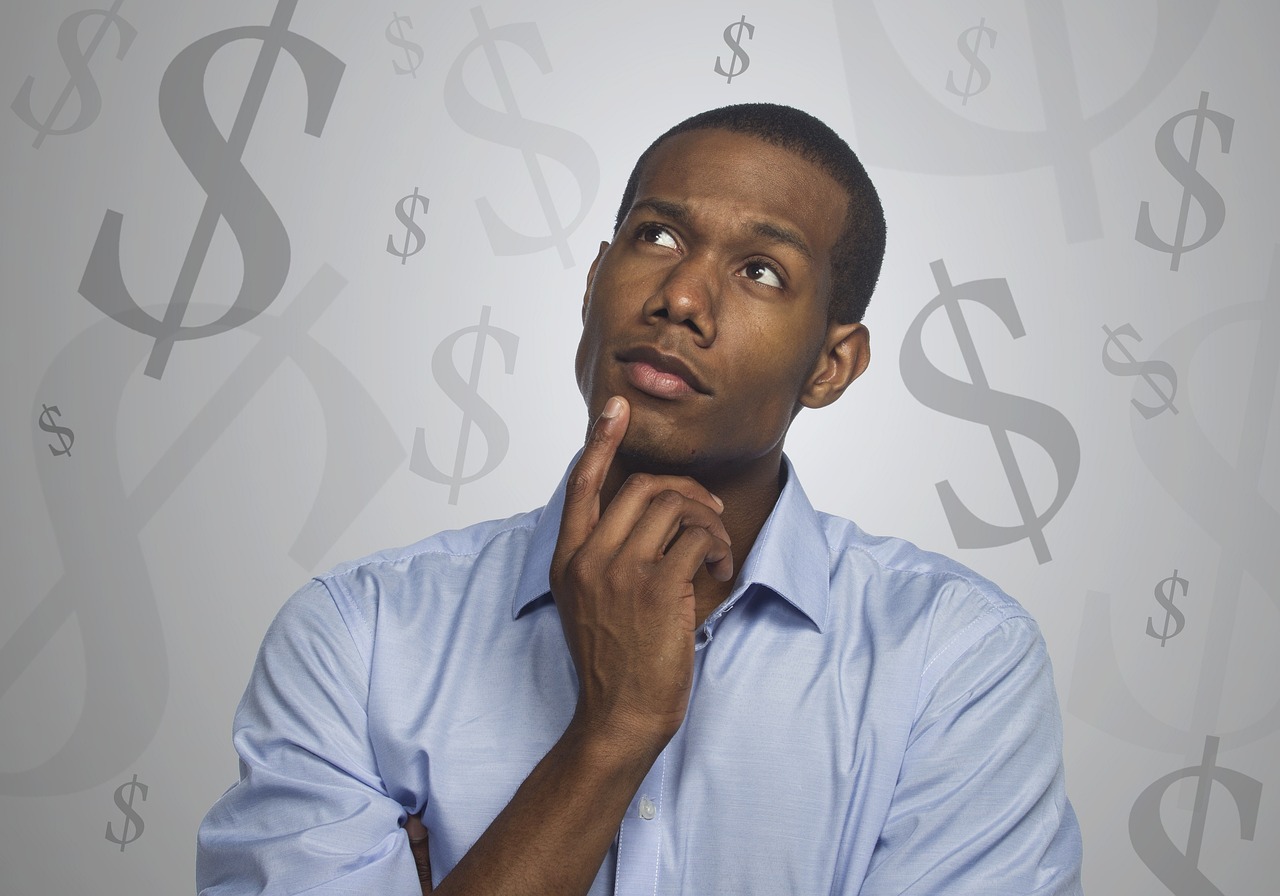
Average BCBA Salary In New Jersey
July 10, 2025
Exploring BCBA Earnings: New Jersey's Salary Landscape

U.S. Pharmaceutical Statistics
July 10, 2025
Navigating the Dynamics of the U.S. Pharmaceutical Market
We’re here to help you
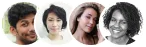
Our team is here to assist you in this process. Contact us for any assistance.
it’s easy to apply
Most commercial insurances accepted
Contact us for any questions regarding coverage or plans – we’ll be happy to provide you with the clearest guidance as to your best options.